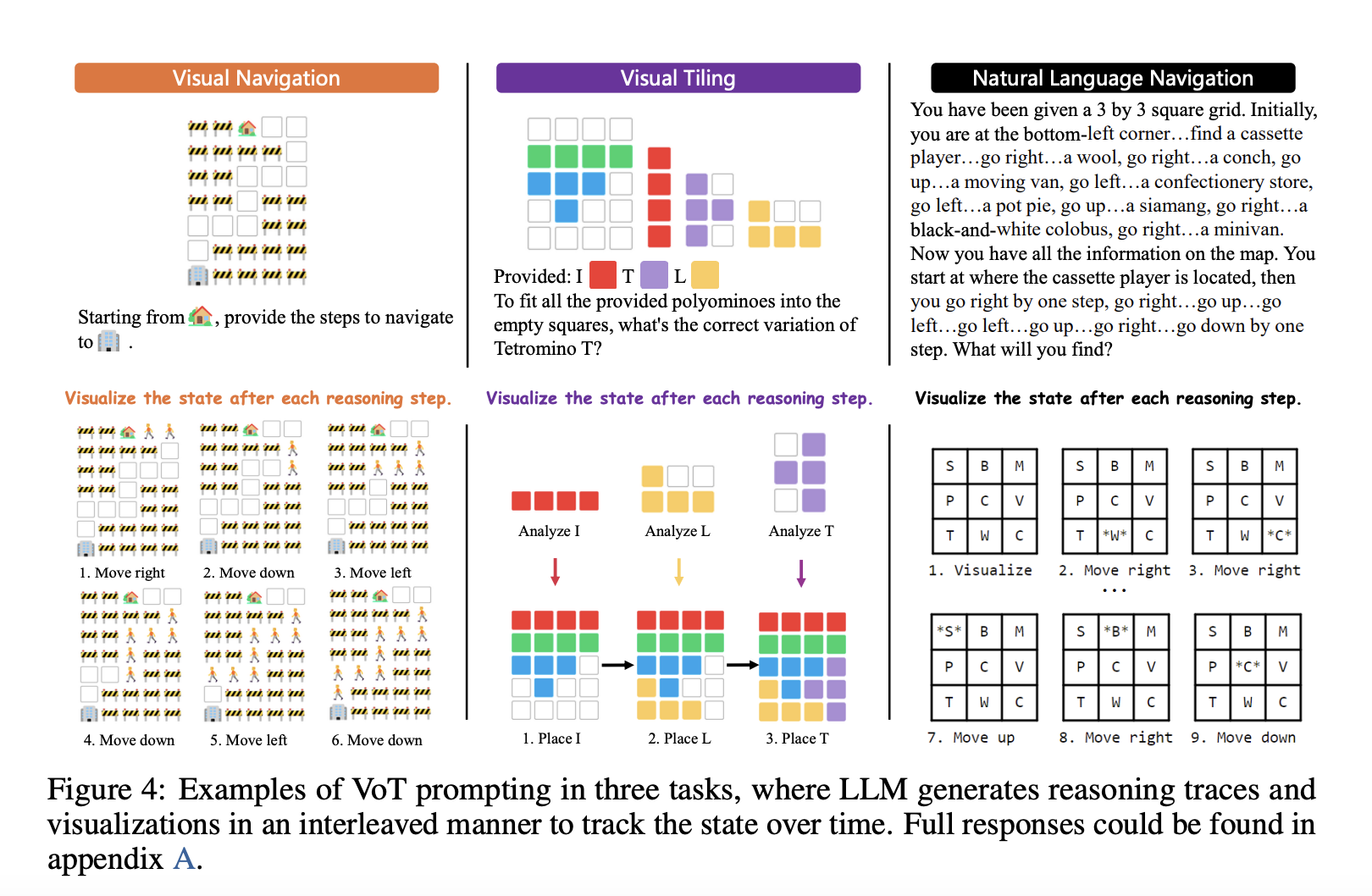
Large language models (LLMs) excel in language comprehension and reasoning tasks but lack spatial reasoning exploration, a vital aspect of human cognition. Humans demonstrate remarkable skills in mental imagery, termed the Mind’s Eye, enabling imagination of the unseen world. This capability remains relatively unexplored in LLMs, highlighting a gap in their understanding of spatial concepts and their inability to replicate human-like imagination.
Previous studies have highlighted the remarkable achievements of LLMs in language tasks but underscored their underexplored spatial reasoning abilities. While human cognition relies on spatial reasoning for environmental interaction, LLMs primarily depend on verbal reasoning. Humans augment spatial awareness through mental imagery, enabling tasks like navigation and mental stimulation, a concept extensively studied across neuroscience, philosophy, and cognitive science.
Microsoft researchers propose Visualization-of-Thought (VoT) prompting. It can generate and manipulate mental images similar to the human mind’s eye for spatial reasoning. Through VoT prompting, LLMs utilise a visuospatial sketchpad to visualise reasoning steps, enhancing subsequent spatial reasoning. VoT employs zero-shot prompting, utilising LLMs’ capability to acquire mental images from text-based visual art, instead of relying on few-shot demonstrations or text-to-image techniques with CLIP.
VoT prompts LLMs to generate visualisations after each reasoning step, forming interleaved reasoning traces. Utilising a visuospatial sketchpad tracks the visual state, represented by partial solutions at each step. This mechanism grounds LLMs’ reasoning in the visual context, improving their spatial reasoning abilities within tasks like navigation and tiling.
GPT-4 VoT surpasses other settings across all tasks and metrics, indicating the effectiveness of visual state tracking. Comparisons reveal significant performance gaps, highlighting VoT’s superiority. In the natural language navigation task, GPT-4 VoT outperforms GPT-4 w/o VoT by 27%. Notably, GPT-4 CoT lags behind GPT-4V CoT in visual tasks, suggesting the advantage of grounding LLMs with a 2D grid for spatial reasoning.
The key contributions of this research are the following:
- The paper explores LLMs’ mental imagery for spatial reasoning, analysing its nature and constraints while delving into its origin from code pre-training.
- It introduces two unique tasks, “visual navigation” and “visual tiling,” accompanied by synthetic datasets. These offer diverse sensory inputs for LLMs and varying complexity levels, thereby providing a robust testbed for spatial reasoning research.
- The researchers propose VoT prompting, which effectively elicits LLMs’ mental imagery for spatial reasoning, showcasing superior performance compared to other prompting methods and existing multimodal large language models (MLLMs). This capability resembles the human mind’s eye process, implying its potential applicability in enhancing MLLMs.
In conclusion, the research introduces VoT, which mirrors human cognitive function in visualising mental images. VoT empowers LLMs to excel in multi-hop spatial reasoning tasks, surpassing MLLMs in visual tasks. Similar to the mind’s eye process, this capability indicates promise for MLLMs. The findings underscore VoT’s efficacy in enhancing spatial reasoning in LLMs, suggesting its potential to advance multimodal language models.
Check out the Paper. All credit for this research goes to the researchers of this project. Also, don’t forget to follow us on Twitter. Join our Telegram Channel, Discord Channel, and LinkedIn Group.
If you like our work, you will love our newsletter..
Don’t Forget to join our 40k+ ML SubReddit