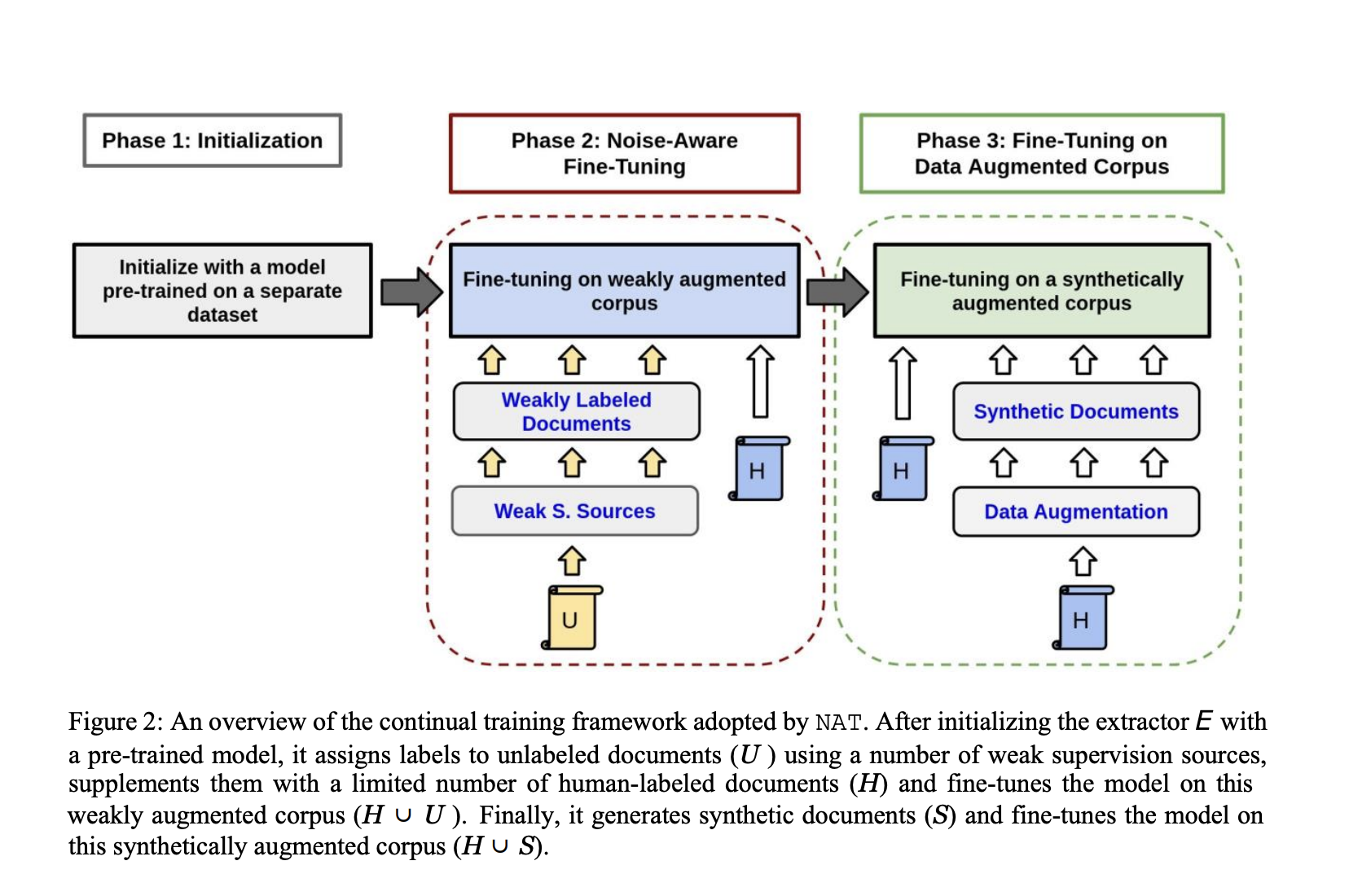
In document processing, particularly visually rich documents (VRDs), the need for efficient information extraction (IE) has become increasingly critical. VRDs, such as invoices, utility bills, and insurance quotes, are ubiquitous in business workflows, often presenting similar information in varying layouts and formats. Automating the extraction of pertinent data from these documents can significantly reduce the manual effort required for parsing. However, achieving a generalizable solution for IE from VRDs poses significant challenges, as it necessitates understanding the document’s textual and visual properties, which cannot be easily retrieved from other sources.
Numerous approaches have been proposed to tackle the task of IE from VRDs, ranging from segmentation algorithms to deep learning architectures that encode visual and textual context. However, many of these methods rely on supervised learning, requiring many human-labeled samples for training.
Labeling highly accurate VRDs is labor-intensive and costly, posing a bottleneck in enterprise scenarios where custom extractors must be trained for thousands of document types. Researchers have turned to pre-training strategies to address this challenge, leveraging unsupervised multimodal objectives to train extractor models on unlabeled instances before fine-tuning on human-labeled samples.
Despite the promise of pre-training strategies, they often require significant time and computational resources, making them impractical in constrained training time. In response to this challenge, a team of researchers from Google AI proposed a semi-supervised continual training method to train robust extractors with limited human-labeled samples within a bounded time. The team Proposed a Noise-Aware Training method or NAT. Their method operates in three phases, leveraging labeled and unlabeled data to iteratively improve the performance of the extractor while respecting the time constraints imposed on training.
The research question at the heart of their study is crucial for advancing the field of document processing, particularly in enterprise settings where scalability and efficiency are paramount concerns. The challenge is to develop techniques that allow for the effective extraction of information from VRDs with limited labeled data and bounded training time. Their proposed method aims to address this challenge, with the ultimate goal of democratizing access to advanced document processing capabilities while minimizing the manual effort and resources required for training custom extractors.
In conclusion, the proposed semi-supervised continual training method not only addresses the challenges inherent in training robust document extractors within strict time constraints but also offers a host of benefits. By leveraging both labeled and unlabeled data systematically, their approach holds the potential to significantly improve the efficiency and scalability of document processing workflows in enterprise environments, ultimately enhancing productivity and reducing operational costs. Their research paves the way for democratizing access to advanced document processing capabilities, marking a significant step forward in the field.
Check out the Paper. All credit for this research goes to the researchers of this project. Also, don’t forget to follow us on Twitter. Join our Telegram Channel, Discord Channel, and LinkedIn Group.
If you like our work, you will love our newsletter..
Don’t Forget to join our 39k+ ML SubReddit
Arshad is an intern at MarktechPost. He is currently pursuing his Int. MSc Physics from the Indian Institute of Technology Kharagpur. Understanding things to the fundamental level leads to new discoveries which lead to advancement in technology. He is passionate about understanding the nature fundamentally with the help of tools like mathematical models, ML models and AI.