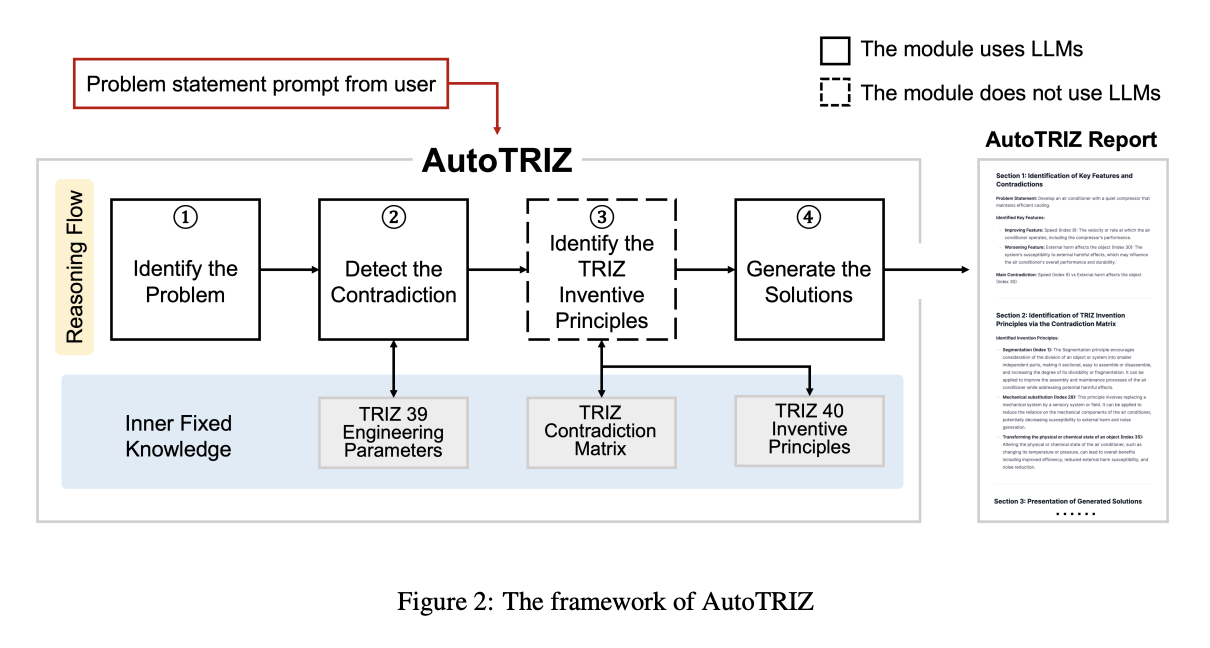
Human designers’ creative ideation for concept generation has been aided by intuitive or structured ideation methods such as brainstorming, morphological analysis, and mind mapping. Among such methods, the Theory of Inventive Problem Solving (TRIZ) is widely adopted for systematic innovation and has become a well-known approach. TRIZ is a knowledge-based ideation methodology that provides a structured framework for engineering problem-solving by identifying and overcoming technical contradictions using inventive principles derived from a large-scale patent database.
Recent advancements integrate machine learning and natural language processing with TRIZ to streamline its reasoning process. Systems like PAT-ANALYZER and PaTRIZ automatically extract contradictory information from patent texts. Some other methods employ text-mining techniques for inventive problem formulation or map TRIZ principles to patents using topic modeling. However, most of these works utilize algorithms to improve specific steps of the TRIZ process. These methods still demand significant user reasoning.
Researchers from the Singapore University of Technology and Design and the City University of Hong Kong present AutoTRIZ, an artificial ideation tool that utilizes LLMs to automate and improve the TRIZ methodology. By harnessing LLMs’ extensive knowledge and advanced reasoning capabilities, AutoTRIZ offers a new approach to design automation and interpretable ideation with artificial intelligence. It generates solutions for user-provided problem statements, adhering to the TRIZ thinking flow and reasoning process.
AutoTRIZ begins with a user-provided problem statement and conducts a four-step reasoning process based on TRIZ principles. It generates a detailed solution report outlining the reasoning process and proposed solutions. The system utilizes a fixed knowledge base segmented into three TRIZ-related segments to guide controlled reasoning. AutoTRIZ emphasizes controlling the problem-solving process while drawing problem-related knowledge from the pre-trained large-scale corpora used to train the LLM.
AutoTRIZ’s detection results were compared with human experts’ analyses from textbooks categorized into complete match, half match, and no match scenarios. While human expert analysis carries subjectivity and bias, it serves as a benchmark for comparison. Results indicate that in 7 out of 10 cases, AutoTRIZ’s top 3 detections completely or partially matched the textbook analyses, demonstrating a degree of overlap between AutoTRIZ and human expert results.
In conclusion, The research introduces AutoTRIZ, an artificial ideation tool that employs LLMs to automate and enhance the TRIZ methodology. Through three LLM-based reasoning modules and a pre-defined function module interacting with a fixed knowledge base, AutoTRIZ generates interpretable solution reports from user-provided problem statements. The method’s effectiveness is demonstrated through quantitative experiments and case studies, suggesting potential extensions to other knowledge-based ideation methods beyond TRIZ.
Check out the Paper. All credit for this research goes to the researchers of this project. Also, don’t forget to follow us on Twitter. Join our Telegram Channel, Discord Channel, and LinkedIn Group.
If you like our work, you will love our newsletter..
Don’t Forget to join our 39k+ ML SubReddit