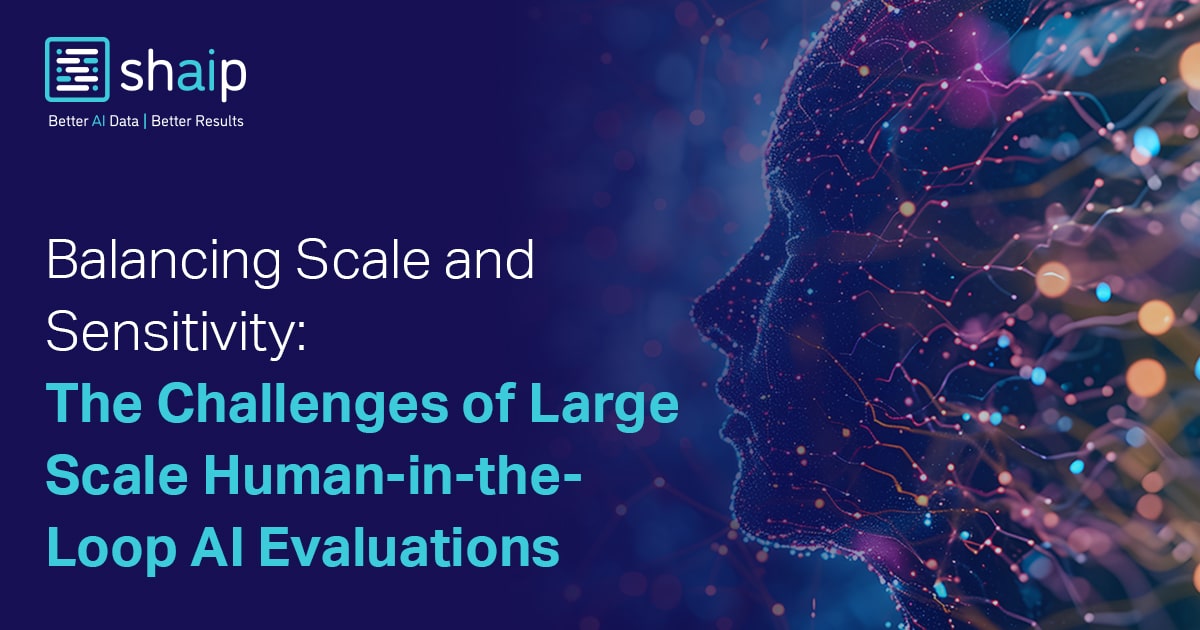
In the rapidly advancing field of artificial intelligence (AI), human-in-the-loop (HITL) evaluations serve as a crucial bridge between human sensitivity and machine efficiency. However, as AI applications scale to accommodate global needs, maintaining the balance between the scale of evaluations and the sensitivity required for accurate outcomes presents a unique set of challenges. This blog explores the intricacies of scaling HITL AI evaluations and offers strategies to navigate these challenges effectively.
The Importance of Sensitivity in HITL Evaluations
At the heart of HITL evaluations lies the need for sensitivity — the ability to accurately interpret and respond to nuanced data that AI alone might misinterpret. This sensitivity is paramount in fields such as healthcare diagnostics, content moderation, and customer service, where understanding context, emotion, and subtle cues is essential. However, as the demand for AI applications grows, so does the complexity of maintaining this level of sensitivity at scale.
Challenges of Scaling HITL AI Evaluations
- Maintaining Quality of Human Feedback: As the number of evaluations increases, ensuring consistent, high-quality feedback from a larger pool of evaluators becomes challenging.
- Cost and Logistical Constraints: Scaling HITL systems requires significant investment in recruitment, training, and management of human evaluators, alongside the technological infrastructure to support them.
- Data Privacy and Security: With larger datasets and more human involvement, ensuring data privacy and protecting sensitive information becomes increasingly complex.
- Balancing Speed and Accuracy: Achieving a balance between the quick turnaround times necessary for AI development and the thoroughness required for sensitive evaluations.
Strategies for Effective Scaling
- Leveraging Crowdsourcing with Expert Oversight: Combining crowdsourced feedback for scalability with expert review for quality control can maintain sensitivity while managing costs.
- Implementing Tiered Evaluation Systems: Using a tiered approach where initial evaluations are performed at a broader level, followed by more detailed reviews for complex cases, can help balance speed and sensitivity.
- Utilizing Advanced Technologies for Support: AI and machine learning tools can assist human evaluators by pre-filtering data, highlighting potential issues, and automating routine tasks, allowing humans to focus on areas requiring sensitivity.
- Fostering a Culture of Continuous Learning: Providing ongoing training and feedback to evaluators ensures that the quality of human input remains high, even as the scale increases.
Success Stories
1. Success Story: Global Language Translation Service
Background: A leading global language translation service faced the challenge of maintaining the quality and cultural sensitivity of translations across hundreds of language pairs at a scale required to serve its worldwide user base.
Solution: The company implemented a HITL system that combined AI with a vast network of bilingual speakers worldwide. These human evaluators were organized into specialized teams according to linguistic and cultural expertise, tasked with reviewing and providing feedback on AI-generated translations.
Outcome: The integration of nuanced human feedback significantly improved the accuracy and cultural appropriateness of translations, enhancing user satisfaction and trust in the service. The approach allowed the service to scale efficiently, handling millions of translation requests daily without compromising quality.
2. Success Story: Personalized Learning Platform
Background: An educational technology startup developed an AI-driven personalized learning platform that aimed to adapt to the unique learning styles and needs of students across various subjects. The challenge was ensuring the AI’s recommendations remained sensitive and appropriate for a diverse student population.
Solution: The startup established a HITL evaluation system where educators reviewed and adjusted the AI’s learning path recommendations. This feedback loop was supported by a dashboard that allowed educators to easily provide insights based on their professional judgment and understanding of students’ needs.
Outcome: The platform achieved remarkable success in personalizing learning at scale, with significant improvements in student engagement and performance. The HITL system ensured that AI recommendations were both pedagogically sound and personally relevant, leading to widespread adoption in schools.
3. Success Story: E-commerce Customer Experience
Background: An e-commerce giant sought to improve its customer service chatbot’s ability to handle complex, sensitive customer issues without escalating them to human agents.
Solution: The company leveraged a large-scale HITL system where customer service representatives provided feedback on chatbot interactions. This feedback informed continuous improvements in the AI’s natural language processing and empathy algorithms, enabling it to better understand and respond to nuanced customer queries.
Outcome: The enhanced chatbot significantly reduced the need for human intervention while improving customer satisfaction rates. The success of this initiative led to the chatbot’s expanded use across multiple customer service scenarios, demonstrating the effectiveness of HITL in refining AI capabilities.
4. Success Story: Health Monitoring Wearable
Background: A health tech company developed a wearable device designed to monitor vital signs and predict potential health issues. The challenge was to ensure the AI’s predictions were accurate across a diverse user base with varying health conditions.
Solution: The company incorporated HITL feedback from healthcare professionals who reviewed the AI’s health alerts and predictions. This process was facilitated by a proprietary platform that streamlined the review process and allowed for rapid iteration of the AI algorithms based on medical expertise.
Outcome: The wearable device became known for its accuracy and reliability in predicting health events, significantly improving patient outcomes and preventive care. The HITL feedback loop was instrumental in achieving a high level of sensitivity and specificity in the AI’s predictions, leading to its adoption by healthcare providers worldwide.
These success stories exemplify the transformative potential of incorporating human feedback into AI evaluation processes, especially at scale. By prioritizing sensitivity and leveraging human expertise, organizations can navigate the challenges of large-scale HITL evaluations, leading to innovative solutions that are both effective and empathetic.
Conclusion
Balancing the scale and sensitivity in large-scale HITL AI evaluations is a complex, yet surmountable challenge. By strategically combining human insights with technological advancements, organizations can scale their AI evaluation efforts effectively. As we continue to navigate this evolving landscape, the key lies in valuing and integrating human sensitivity at every step, ensuring that AI development remains both innovative and empathetically grounded.
Discover more from reviewer4you.com
Subscribe to get the latest posts to your email.