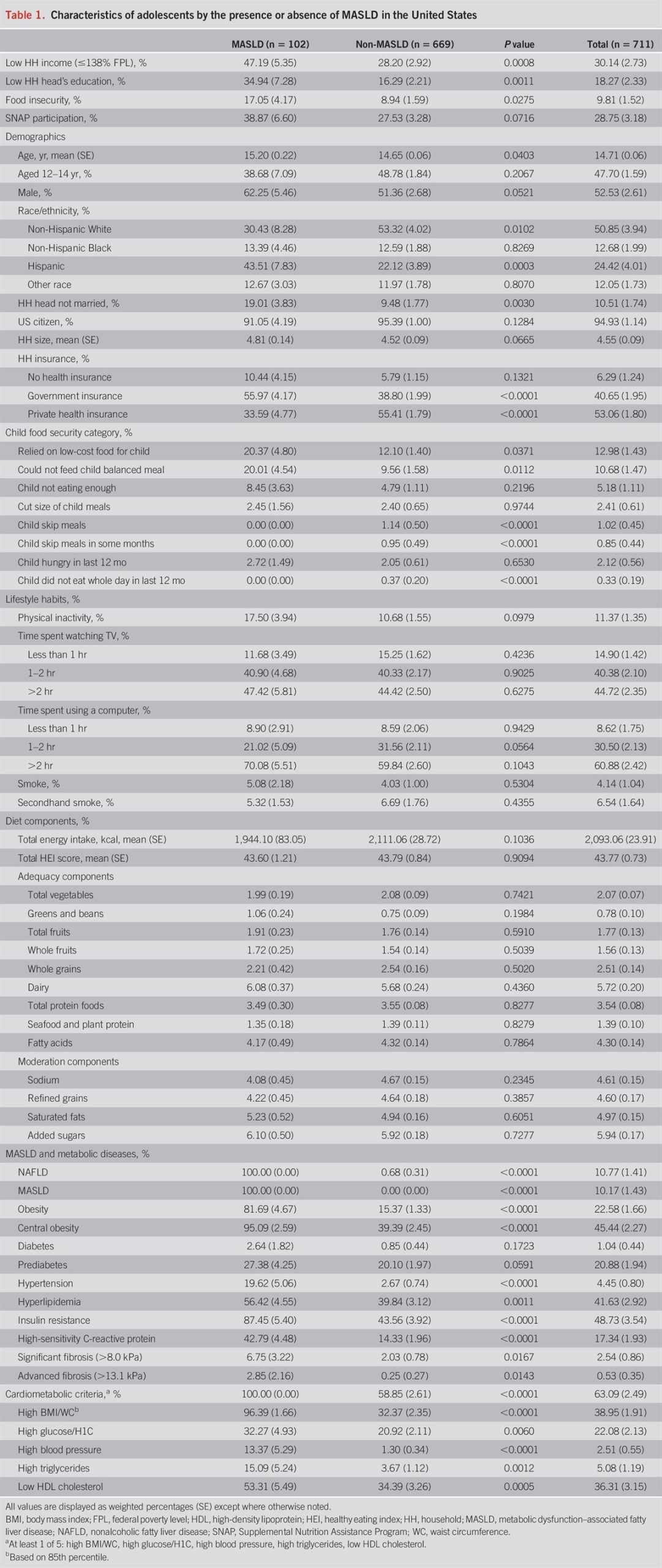
INTRODUCTION
Metabolic dysfunction–associated fatty liver disease (MASLD) is the most common cause of chronic liver disease (CLD), which is driven by environmental factors (unhealthy food, lack of physical activity, and environmental toxins) and potentially genetic predisposition (1–10). In this context, MASLD is also closely associated with the metabolic conditions of obesity, type 2 diabetes (T2D), dyslipidemia, and hypertension (HTN) such that the higher the number of metabolic comorbidities present, the higher the risk of mortality (11,12). The global prevalence of MASLD has surged to 38% among adults over the past decade (13). There is significant evidence to suggest that the burden of MASLD is also increasing among children and adolescents (1,3,14). In 2006, the prevalence of MASLD among those aged 2–19 years in the United States was estimated at 9.6% with rates ranging from 0.7% in younger children (ages 2–4 years) to 17.3% in those aged 15–19 years. The prevalence rates vary by ethnicity, reported as 10.2% for Asian, 1.5% for Blacks, 11.8% for Hispanics, and 8.6% for White individuals (15). Another study reported that the incidence of MASLD among children increased by 62% between 2009 and 2018 (36.0 per 100,000 in 2009 to 58.2 per 100,000 in 2018), noted to be significantly higher in children of Hispanic ethnicity when compared with that in the general population (78.3% vs 43.3%; P < 0.0001) (14).
The prevalence of MASLD is dramatically affected by the presence and level of obesity where MASLD prevalence is reported to be in 26% (29.4% in male; 22.6% in female) among children and adolescents with obesity reaching 58% among the morbid obese who underwent bariatric surgery and had a liver biopsy (16,17). Although the pathophysiology of MASLD among younger individuals is different than that among adults (18–20), treatment of MASLD in children and adolescents is similar to those recommended for adults, where a healthy diet and appropriate levels of exercise are the first line of intervention (21,22). However, during childhood and adolescence, eating a healthy diet may be dependent upon one’s caretakers’ ability to provide appropriate nutrition. As such, food insecurity defined as the ability to acquire adequate food for active, healthy living is limited by a lack of money and other resources, may play a role in the development of MASLD among adolescents. However, although there are data reporting the association of food insecurity and obesity among adults, such data are lacking for the younger population (23–28). Therefore, the purpose of this study was to investigate the associations between socioeconomic factors and MASLD among adolescents (aged 12–18 years) in the United States.
METHODS
Study population
We performed a cross-sectional study that used data from the National Health and Nutrition Examination Survey (NHANES) 2017–2018. The NHANES is a periodic, cross-sectional sampling survey conducted by the National Center for Health Statistics, a unit of the Centers for Disease Control and Prevention whose purpose is to monitor the health and nutritional status of civilian, noninstitutionalized subjects in the US population on 2-year cycles. Survey data include disease presence/absence, disease risk factors, and nutritional status and are collected through household (HH) interviews and standardized medical examinations, including through blood and urine samples collected in mobile examination centers. Detailed descriptions of the plan and operation of the NHANES have been previously published (29). In our analysis, we included adolescents between 12 and 18 years of age with a completed HH food security questionnaire and liver ultrasound transient elastography (TE) examination. Because this study used a publicly available data set and the data are deidentified according to 45 CFR 46,102, this research is not considered human subjects research so does not require an institutional review board review. Nevertheless, this study was reviewed and granted exemption by the Inova Institutional Review Board and followed the Strengthening the Reporting of Observational Studies in Epidemiology reporting guidelines for cohort studies.
Definition of socioeconomic status, education level, and food insecurity
For each adolescent who participated in the survey, a responsible adult living at home provided information on demographics, including family size and income, and health insurance status. The poverty income ratio was calculated by dividing family income by the poverty guidelines (30). Low HH income was defined as a poverty income ratio of <138% of the federal poverty level. In the United States, among people with low incomes, there are multiple government and state-funded assistance programs to help individuals and families meet their basic needs. The Supplemental Nutrition Assistance Program (SNAP) is one such program that is designed to provide each family member with a certain amount of money each month that is to be used to buy food by supplementing one’s income (31). The SNAP is complex as the amount received is based on HH size and HH income. The money is provided in the form of a debit card and can only be used to buy food that is designated as healthy food as determined by the US Department of Agriculture healthy eating guidelines and recommended daily allowances. For this study, SNAP participation was defined as anyone in the HH having participated within the past 12 months. Adolescents were classified as income-eligible SNAP nonparticipants if they did not participate in SNAP but lived with a low HH income that would qualify for SNAP.
The head of the HH was defined as the person who owned or rented the home in which the adolescent lived. Low head of HH education level was defined as having less than a high school education.
The NHANES assesses adolescent food security through the US Department of Agriculture Child Food Security Survey Module (32), which includes 8 questions (see Supplementary Table 1, Supplementary Digital Content 2, https://links.lww.com/AJG/D217). Adolescents with 2 or more affirmative survey responses are classified as having food insufficiency (low or very low food security).
Dietary Assessment and Healthy Eating Index 2015
We merged 24-hour dietary recall of 2-day data from the NHANES with US Department of Agriculture Food Patterns Equivalents data for 2017–2018 to create the Healthy Eating Index 2015 (HEI-2015) food groups (33). The HEI-2015 consists of 13 components, including 9 dietary adequacy ones (total fruits, whole fruits, total vegetables, greens and beans, whole grains, dairy, total protein foods, seafood and plant proteins, and fatty acid ratio), with higher scores indicating higher consumption, and 4 moderation ones (refined grains, sodium, added sugars, and saturated fats), with higher scores indicating lower consumption (see Supplementary Table 2, Supplementary Digital Content 2, https://links.lww.com/AJG/D217). The maximum score for total fruits, whole fruits, total vegetables, greens and beans, dairy, total protein foods, and seafood and plant proteins is 5 points, and for the other components, 10 points. The total HEI-2015 score ranges from 0 to 100, with higher scores indicating superior diet quality, and was calculated by adding the 13 component scores. Total energy intake (kilocalories per day) was also calculated, using NHANES dietary interview data.
Definitions of MASLD, metabolic risk factors, and others
For the first time in the NHANES survey process, NHANES 2017–2018 used vibration-controlled TE to estimate hepatic fibrosis by measuring liver stiffness and quantifying liver fat using the controlled attenuation parameter (CAP). Elastography has been extensively evaluated for its accuracy in assessing liver steatosis and fibrosis (34). Liver stiffness measurements range from 1.5 to 75 kPa, with higher values indicating more severe fibrosis. CAP values range from 100 to 400 dB/m, with higher values indicating higher amounts of liver fat. Nonalcoholic fatty liver disease (NAFLD) was diagnosed by using a median CAP score of ≥285 dB/m and an optimized sensitivity of 80% and specificity of 77% for detecting hepatic steatosis (35), in the absence of any other possible causes of CLD (36). Excessive alcohol use was not excluded because alcohol use in adolescents has not been publicly published. MASLD was defined as having NAFLD with the presence of a designated cardiometabolic risk factor. Cardiometabolic risk factors for adolescents were defined as the following: (i) BMI ≥85th percentile for age/sex or waist circumference ≥95th percentile; (ii) fasting serum glucose of ≥100 mg/dL, serum glucose of ≥200 mg/dL or hemoglobin A1c (HbA1c) of ≥5.7% or T2D or treatment for T2D; (iii) blood pressure ≥130/85 mm Hg or specific antihypertensive drug treatment; (iv) plasma triglycerides ≥150 mg/dL or lipid-lowering treatment; and (v) plasma high-density lipoprotein cholesterol <40 mg/dL or lipid-lowering treatment. Significant fibrosis and advanced fibrosis (AF) were defined as a liver stiffness of ≥8.0 and ≥13.1 kPa, respectively (37).
Obesity in adolescents was defined as a body mass index (BMI) at or above the 95th percentile for sex and age, according to the 2000 Centers for Disease Control and Prevention growth charts for the United States. Central obesity was defined by age-sex–specific waist circumference (38,39). T2D was defined as a fasting glucose level of ≥126 mg/dL, HbA1c of ≥6.0%, self-reported medical history of T2D, or oral hypoglycemic agent or insulin use. Prediabetes was defined as a fasting plasma glucose value of 100–125 mg/dL or HbA1c level between 5.7% and 6.4%, in the absence of an established T2D diagnosis. HTN was defined as having a systolic or diastolic blood pressure of >140 mm Hg and >90 mm Hg, respectively, from an average of 3 measurements, or a history of high blood pressure, or a history of oral antihypertensive medication use. Hyperlipidemia (HL) was defined as a serum cholesterol level of ≥200 mg/dL, low-density lipoprotein level of ≥130 mg/dL, and high-density lipoprotein level of ≤40 mg/dL in men or ≤50 mg/dL in women. A high C-reactive protein (CRP) level was defined as a CRP >2 mg/L. Insulin resistance was defined as homeostasis model assessment of insulin resistance level of ≥2.5. Information on demographic characteristics (i.e., age, sex, and race/ethnicity) and lifestyle (i.e., smoking status, secondhand smoke, physical activity, and time spent watching TV or computer) was collected at baseline through self-reports. Physical inactivity was defined as 0 days of being physically active at least 60 minutes per week.
Statistical analysis
Examination sample weights, accounting for nonresponse, noncoverage, and unequal selection probabilities for certain categories of the population, were incorporated to produce national estimates for all analyses. Sampling errors were estimated by the Taylor series linearization method (40). Differences in sociodemographic and clinical characteristics and dietary components between comparison groups were tested by using a t statistic for continuous variables and the Rao-Scott χ2 test for categorical variables. Multivariable logistic regression models were used to measure the association of each socioeconomic status with MASLD. Multivariable models were constructed in several stages, including age-sex-race–adjusted ones and important metabolic abnormalities, including obesity, HTN, HL, T2D, prediabetes, insulin resistance, and high CRP level-adjusted ones. To maintain sufficient power in the models, only significant metabolic abnormalities were included in the fully adjusted models.
Sensitivity analyses were conducted by using a median CAP score of ≥263 dB/m for detecting the presence of 5% steatosis (41). All analyses were performed using survey procedures (SAS, version 9.4; SAS Institute, Cary, NC) to account for the NHANES complex sample design. A 2-sided P < 0.05 was considered the threshold for statistically significant.
RESULTS
Of 1,051 adolescents from the NHANES, 1,003 (95.4%) underwent an examination at a mobile examination center. Of these subjects, 215 were excluded due to lack of food security survey or TE examination. Another 17 subjects were excluded due to having viral hepatitis, leaving a final analytic sample of 771 adolescents.
Overall population characteristics
Among the 771 adolescents included in our analysis (mean age 14.7 years; 52.5% male; 50.9% White, 12.7% Black, 24.4% Hispanic, and 12.0% Other), 9.8% were food insecure, 30.1% had a family income below the poverty threshold, 18.3% lived with a head of HH with a low education level, 28.8% participated in SNAP, 22.6% were obese, 45.4% had central obesity, 1.0% had T2D, 20.9% had prediabetes, 4.5% had HTN, 41.6% had HL, 17.3% had high CRP levels, and 63.1% had ≥1 cardiometabolic criteria. Furthermore, their mean HEI-2015 score was 43.8%, and 11.4% were considered physically inactive (Table 1). MASLD affects approximately 4.27 million adolescents with the prevalence of 10.12% (95% confidence interval [CI] 7.13%–13.20%) (42). By contrast, NAFLD affects approximately 4.52 million with the prevalence of 10.77% (95% CI 7.76–13.78). There was near-perfect concordance between MASLD and NAFLD (Cohen κ coefficient of 0.971, 95% CI 0.946–0.996).

Characteristics of adolescents by the presence or absence of MASLD in the United States
Sociodemographic characteristics by MASLD status
Sociodemographic characteristics of adolescents by the presence or absence of MASLD are summarized in Table 1. Compared with adolescents without MASLD, those with MASLD were more likely to live in a food-insecure HH (17.1% vs 8.9%), with a low HH income (47.2% vs 28.2%), with a head of HH with a low education level (34.9% vs 16.3%), and have a single head of HH (19.0% vs 9.5%) (P < 0.03). In addition, they relied more on low-cost food (20.4% vs 12.1%) and did not eat balanced meals (20.0% vs 9.6%) (P < 0.04). Furthermore, they were slightly older (15.2 years vs 14.7 years) and more likely to be Hispanic (43.5% vs 22.2%) and had higher rates of obesity (81.7% vs 15.4%), central obesity (95.1% vs 39.4%), HTN (19.6% vs 2.7%), HL (56.4% vs 39.8%), prediabetes (27.4% vs 20.1%), insulin resistance (87.5% vs 43.6%), high CRP levels (42.8% vs 14.3%), significant fibrosis (6.8% vs 2.0%), and AF (2.9% vs 0.3%) (P < 0.02). There were no significant differences among adolescents according to MASLD status in those who were physically inactive, the amount of time spent watching TV or using the computer, or the HEI-2015 mean score and its 13 components.
Sociodemographic characteristics and MASLD prevalence by socioeconomic status
In comparison with food-secure adolescents, food-insecure ones were more likely to live with a low HH income (70.4% vs 25.7%) and a head of HH with a low education level (29.2% vs 17.0%) (P < 0.04) (Table 2). Low HH income or head of HH education level adolescents were also significantly more likely to be food-insecure with compared with higher HH income or head of HH education level ones (23.2% vs 4.2% and 16.3% vs 8.8%, respectively) (P < 0.04). Compared with their counterparts, food-insecure, low HH income, and low head of HH education level adolescents were more likely to participate in SNAP (62.5% vs 25.1%, 61.9% vs 14.9%, and 42.0% vs 26.2%, respectively), be non-US citizens (95.6% vs 88.8%, 98.0% vs 90.0%, and 97.8% vs 82.1%, respectively), have government health insurance (75.9% vs 36.9%, 73.4% vs 25.2%, and 64.1% vs 35.2%, respectively), live in single head HH (25.2% vs 8.9%, 17.9% vs 6.0%, and 18.5% vs 9.2%, respectively), and rely on low-cost food (98.7% vs 3.8%, 31.9% vs 5.2%, and 21.7% vs 11.7%, respectively) and less likely to have balanced meals as defined by food security questions (93.2% vs 3.8%, 23.3% vs 5.5%, and 16.5% vs 9.9%, respectively) (P < 0.05) (Table 2).

Characteristics of adolescents by socioeconomic status in the United States
Comparing rates of metabolic abnormalities by socioeconomic status, adolescents who lived with a low HH income had higher rates of obesity (31.5% vs 18.4%), central obesity (58.1% vs 39.6%), HL (46.8% vs 37.6%), insulin resistance (60.0% vs 44.1%), and high CRP levels (26.7% vs 13.2%) (P < 0.01) compared with adolescents with a higher HH income. Compared with their counterparts, adolescents who lived with a head of HH with a low education level had higher rates of high CRP levels (24.3% vs 15.2%) (P < 0.01). There were no differences in metabolic disease rates, including T2D, according to food insecurity. In terms of lifestyle factors, there were no significant differences in self-reported exercise levels and smoking or exposure to secondhand smoke status based on socioeconomic status.
The prevalence of MASLD (Table 2) was greater among food-insecure adolescents vs food-secure ones (17.4% vs 9.4%) and adolescents living with a low HH income vs a higher HH income (15.0% vs 7.2%) and living with a head of HH with a lower education level vs a higher education level (18.0% vs 8.2%) (P < 0.05).
Multivariable association of food insecurity, low HH income, and low head of HH education level and MASLD risk
Logistic regression models were performed to determine whether being food insecure, from a low-income HH, or from a HH where the head had a low education level were associated with MASLD, after adjustment for potential confounders (Table 3). In the age-sex-race–adjusted model, food-insecure and low HH income adolescents were at 137% (odds ratio [OR] 2.37, 95% CI 1.24–4.53) and 107% (OR 2.04, 95% CI 1.26–3.40) greater risk of having MASLD when compared with their counterparts. There was no significant risk of having MASLD when living in a home where the HH head had a low education level or whether they participated in SNAP. In the demographic, BMI, and HTN-adjusted model, the association between food insecurity and MASLD persisted (OR 2.36, 95% CI 1.09–5.10), but the association between low HH income and MASLD was attenuated (P = 0.086).

Food insecurity, low Household income, and low household head’s education association with MASLD among adolescents
Given these results, we assessed for an interaction effect between food insecurity and low HH income on MASLD risk, which was found to be significant. By contrast, there was no significant interaction between food insecurity and low head of HH education level. Compared with adolescents living with a higher HH income, those living with a low HH income with food insecurity had a 3-fold greater risk (OR 3.25, 95% CI 1.31–8.08) of having MASLD, while food-secure adolescents living in low-income HH had no increased risk (OR 1.58, 0.85–2.93, P = 0.139). The fully adjusted odds of having MASLD was elevated by +163% with the presence of HTN (OR 2.63, 1.02–6.78), +241% with being Hispanic (OR 3.41, 1.36–8.56), and +138% with being male (OR 2.38, 1.20–4.75). In addition, a 1-unit increase in BMI was associated with 25% increase in the odds of having MASLD (OR 1.25, 1.17–1.33) among US adolescents (Table 4). These findings remained similar when we used the previous NAFLD definition and when MASLD was defined by a median score of ≥263 dB/m.

Socioeconomic and metabolic risk factors associated with MASLD among adolescents
Subgroup analyses showed that among adolescents living with a low HH income, food insecurity remained a risk factor of having MASLD (OR 2.23, 95% CI 1.14–4.37, P = 0.0228 in the fully adjusted model; MASLD prevalence: food-secure ones 13.8% vs food-insecure ones 23.2%) (see Supplementary Table 3, Supplementary Digital Content 2, https://links.lww.com/AJG/D217). Of note, there were no significant differences in demographics, including socioeconomic status, lifestyle, dietary components, and metabolic abnormalities by food insecurity status, except for sex. In addition, significant differences in MASLD prevalence between food-insecure and food secure ones were found overall (18.72% vs 9.90%, P < 0.05), in 15- to 18-year-old (24.85% vs 11.40%, P = 0.007) and among the girls (20.53% vs 6.93%, P = 0.011). There were no significant differences according to ethnicity (Figure 1). These findings remained similar by NAFLD prevalence (see Supplementary Figure 1, Supplementary Digital Content 1, https://links.lww.com/AJG/D216).

Metabolic dysfunction–associated fatty liver disease prevalence by sex, age, and race among adolescent children in the United States.
Compared with SNAP nonparticipants, income-eligible SNAP participants were more likely to have higher rates of obesity (36.9% vs 22.8%), central obesity (64.7% vs 47.5%), and HL (51.3% vs 39.4%). There were no differences in food insecurity (25.8% vs 18.8%, P = 0.118), MASLD prevalence (21.2% vs 13.2%, P = 0.118), and dietary components as measured by the HEI-2015 (total HEI-2015 score, 43.5 vs 41.9%, P = 0.394) (see Supplementary Table 4, Supplementary Digital Content 2, https://links.lww.com/AJG/D217).
DISCUSSION
This is the first study to investigate the associations between socioeconomic factors and MASLD among adolescents in the United States. In this cohort of 771 adolescents from NHANES 2017–2018, which represents approximately 22.9 million adolescents in the United States, our data show that 1 of 10 adolescents do not get enough food to eat and approximately a third live in a low-income family and participate in the SNAP. Our data also show that adolescents with MASLD are more frequently food insecure, live in a low-income HH where the head of the HH is single and has a lower level of education. Adolescents with MASLD tended to be male, obese, Hispanic, and older than 14 years of age with metabolic risks such as HTN, HL, and high CRP levels. In addition, they were noted to be from HH that relied on low-cost food and lacked the availability of well-balanced meals. Specifically, those most at risk seem to be male individuals with obesity and the metabolic syndrome, Hispanic, and with food insecurity living in a low socioeconomic environment.
Although there is accumulating evidence supporting an association between poor socioeconomic status and an increased risk of cardiometabolic risks among food-insecure adults (39), we did not find that food-insecure adolescents had significantly higher rates of metabolic abnormalities when compared with food-secure adolescents. On the contrary, food-insecure adolescents did have 2.4 times higher odds of having MASLD than food-secure adolescents. This was true even after adjusting for demographic, marital status, and metabolic confounders. Furthermore, our data showed that not having enough food to eat increased the adolescent’s risk of MASLD among low-income families. These data suggest that in adolescents, MASLD may be the predominant manifestation of metabolic abnormality. In addition, our findings support the notion that food insecurity is an independent contributor to the burgeoning prevalence of MASLD among adolescents (23–28).
On the contrary, we were unable to determine how food insecurity among adolescents affected the development of MASLD without a link to metabolic abnormalities. Nevertheless, we believe that the relationship can be due to the quality of food consumed and the lack of physical activity, both factors considered MASLD risks among the adult population (5–8). Although our data did not show any difference in macronutrient consumption based on food security status except for a trend in lower protein food intake, we did show that both food-insecure adolescents and adolescents with MASLD were more likely to rely on low-cost foods, which are probably mostly ultra-processed foods and foods that could be higher in hidden sugar and saturated fats, known to be related to MASLD (43). In addition, many adolescents did not consume balanced meals, all of which are factors that can lead to diet-sensitive comorbidities (44–47). Studies have also shown that children and adolescents who were not consistently fed may undergo periods of food overconsumption followed by periods of underconsumption, which has been shown to lead to obesity and other metabolic diseases in this age cohort (48–55). Unfortunately, these factors may not be captured by self-reported dietary intake (HEI-2015), and this may explain the lack of association between HEI and MASLD in our study.
There was also a lack of difference in the self-reported time spent in physical activity, time spent watching TV, time using a computer, smoking or exposure to secondhand smoke based on food security, or MASLD status among adolescents. However, because most adolescents seem to engage in sedentary behavior (more than 60% spent >1 hour watching TV/per day and 80% spent >1 hour on the computer), a known risk factor of MASLD, we suggest there may be differences in the amount of sedentary behavior self-reported between those with and without MASLD. Nonetheless, the overall prevalence of sedentary behavior is a cause for concern and requires public policy interventions to address reasons for inadequate physical activity (7,8,44,46,47,56).
We also explored the role of SNAP participation on the prevalence of MASLD among low-income HH. Our study suggests that SNAP participation was not associated with the prevalence of MASLD, and this is most likely due to the fact that most low-income HH in our study participated in SNAP (70% of adolescents from low-income and food-insecure HH and 60% of those from low-income and food-secure HH). Despite lack of association between SNAP and prevalence of MASLD, our analysis showed a higher prevalence of risk factors of MASLD among the low-income SNAP participants. In addition, the SNAP nonparticipants were less likely to participate in other government assistance programs because they may not be aware of SNAP, refuse government assistance, or experience the well-known structural and systemic barriers for participating in government assistance programs. Nonetheless, these data reinforce previous reports suggesting a lack of association between SNAP use and prevalence of obesity (57). In addition, recent studies have indicated that participation in SNAP assists in better health management, including better glucose control; therefore, SNAP could now be considered a tool to promote healthier nutrition for children, adolescents, and adults from low-income HH to help ward off chronic diseases, including MASLD (58,59). This is an especially salient point because SNAP benefits were just updated increasing the allotted money and food items allowed for purchase (SNAP 2021) (58,60).
The main strength of this study was that it used standardized data collection and allowed for generalizability to the national adolescent population in the United States. Specifically, for all our analyses, we used NHANES survey weights to account for the complex, cluster-stratified design of NHANES and allowed for the calculation of nationally representative estimates. In addition, our NAFLD prevalence across groups of interest are reliable estimates with relative SE of less than 30% (61). Thus, we can conclude that our findings are generalized to the national adolescent population in the United States. In addition, in contrast to other NHANES cycles, the current dataset included vibration-controlled TE, thus allowing more accurate diagnosis of MASLD and various stages of fibrosis based on TE. However, there are several limitations to this study as well. First, due to a cross-sectional design of NHANES, temporality and causation cannot be inferred from our analysis. Second, self-reported 24-hour dietary intake is subject to recall or report bias. These biases were limited by using the 2-day 24-hour dietary data and adjusting for total energy in the scoring of the HEI-2015. In addition, the HEI-2015 is a well-validated tool to evaluate healthy dietary patterns in US population and predict mortality (60,62). In addition, unmeasured confounders (in addition to increased intake of poor-quality food as discussed earlier) such as social environmental factors, adverse childhood experiences, hormones, in-utero factors, and the lack of a clear definition of low-cost food could also be associated with both food insecurity and MASLD and may add to the explanation of the current association (63–67). Third, because of the small sample size of AF events, further research is needed to access the association between socioeconomic status and AF. There is also a lack of universal cutoff guidelines for CAP score and liver stiffness among adolescents when using TE. However, we used cutoff points recently validated (41) and then used sensitivity analyses using different cutoff values of CAP suggested for adolescents and obtained similar results, which indicated that our results were robust and consistent. Last, we were unable to obtain alcohol usage for those aged 12–18 years because these data are restricted by the National Center for Health Statistics for protecting the confidentiality of survey subjects. However, based on report of underage drinking, it is very possible that up 50% of MASLD prevalence and more than 12% ≥F2 fibrosis in the older adolescent cohort could be attributable to alcohol consumption resulting in an overestimation of the true prevalence of MASLD among adolescents, highlighting the potential confounding effect of alcohol (68–71). These findings can help to inform the hepatology field as to how the new terminology of metabolic alcohol-related liver disease may need to be incorporated into the assessment of older adolescents with steatotis. Nevertheless, our findings underscore the need for robust new strategies for increasing nutrition and budget management education among food insecure families to reduce the risk of MASLD and the associated AF.
In summary, our study of adolescents in the United States suggests an MASLD prevalence of 11%. In comparison with those without MASLD, male individuals with obesity, those with HTN, and Hispanic living in low-income HH with food insecurity were most at risk of MASLD. Adolescents with MASLD were also less likely to eat a balanced meal and more likely to purchase low-cost food, which may exacerbate the cycle of overeating along with the overconsumption of ultra-processed foods and sugar-sweetened food and beverages, both potential drivers of obesity and T2D. Finally, our study did not find a difference in the prevalence of MASLD by SNAP participation. In fact, in the context of changes (SNAP 2021), SNAP may be considered an interventional tool to help improve the diets of adolescents. However, work must continue to remove the systemic and structural barriers to allow better accessibility to the SNAP and provide more physical activity in school and after-school programs and to develop interventional programs that are age, sex, and culturally appropriate for those at highest risk of MASLD.
CONFLICTS OF INTEREST
Guarantor of article: Zobair M. Younossi, MD, MPH, FACG.
Specific author contributions: Z.M.Y. and J.M.P.: study design. J.M.P.: data collection and data analysis. J.M.P., L.H., and Z.M.Y.: Interpretation of data. J.M.P. and L.H.: drafting of the manuscript. Z.M.Y., J.M.P., L.H., S.Z.-S., and J.V.L.: critical revision of the manuscript for important intellectual content. Z.M.Y.: study supervision. All authors read and approved the final version of the manuscript.
Financial support: Beatty Research Fund and the Center for Outcomes Research in Liver Disease.
Potential competing interests: Z.M.Y. is a consultant or has received research funding from AstraZeneca, Boehringer Ingelheim, Bristol Myers Squibb, Cymabay, GlaxoSmithKline, Intercept, Novo Nordisk, Madrigal, Merck, and Siemens. Z.M.Y. acknowledges grants and speaker fees from AbbVie, Gilead Sciences, MSD, and Roche Diagnostics to his institution, speaker fees from Intercept, Janssen, Novo Nordisk, and ViiV, and consulting fees from Novavax, outside of the submitted work. He also acknowledges support to ISGlobal from the grant CEX2018-000806-S funded by MCIN/AEI/10.13039/501100011033 and the Generalitat de Catalunya through the CERCA Program, outside of the submitted work. All other authors have no conflict of interest to disclose.
Ethics approval and consent to participate: Because this study used a publicly available dataset and the data are deidentified according to 45 CFR 46,102, this research is not considered human subjects research, so does not require an institutional review board review. However, all methods were performed in accordance with relevant guidelines and regulations (Declaration of Helsinki).
Data availability statement: These data were derived from the following resource available in the public domain: https://wwwn.cdc.gov/nchs/nhanes/continuousnhanes/default.aspx?BeginYear=2017.
Study Highlights
WHAT IS KNOWN
- ✓ Recent evidence suggests that food insecurity is a risk factor of the development of metabolic dysfunction–associated fatty liver disease (MASLD) among adults; however, to date, there are no data available on an association of food insecurity and MASLD among adolescents (ages 12–18 years).
WHAT IS NEW HERE
- ✓ Using the National Health and Nutrition Examination Survey (NHANES) data from 2017 to 2018 and data from the children’s food insecurity questionnaire, we found that adolescents from low-income households who experienced food insecurity had a 3-fold higher chance of developing MASLD.
- ✓ Food insecurity and MASLD were most likely the result of eating low-cost food (e.g., ultra-processed foods and sugar sweetened beverages and not having balanced meals).
- ✓ Being male, with obesity, with hypertension, Hispanic, and with food-insecurity living in a low socioeconomic environment is the group at highest risk of MASLD.
- ✓ These findings have implications for school breakfast and lunch programs where emphasis should be placed on serving well-balanced and healthy meals.
- ✓ Healthcare providers can assist families especially low-income families with accessing programs that provide food assistance.
- ✓ The recently revised Supplemental Nutrition Assistance Program in the United States may be an intervention tool to assist families in obtaining and maintaining food security.
- ✓ Structural and race-based barriers need to be addressed so that Supplemental Nutrition Assistance Program benefits can be more easily accessed by those most in need.
REFERENCES
Discover more from reviewer4you.com
Subscribe to get the latest posts to your email.