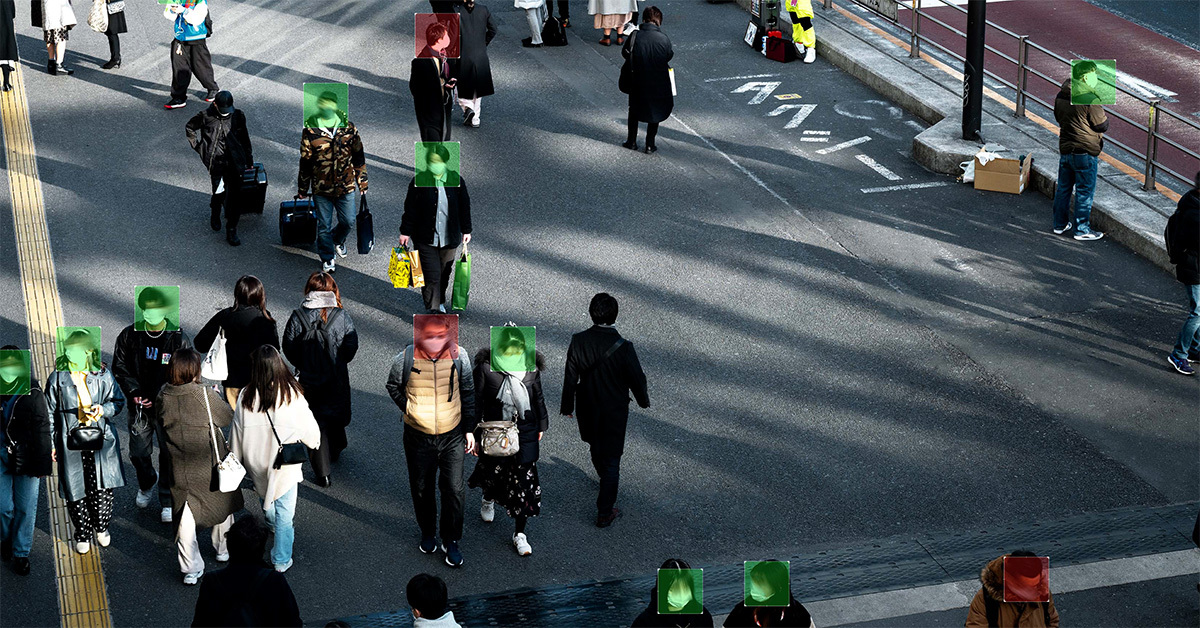
March 28, 2024
4 min read
By Cogito Tech.
17 views
What is Data Annotation?

The assignment of attributes, tags or labels to data to assist machine learning algorithms in comprehending and classifying information that requires processing is known as data annotation.
It plays a vital role in training artificial intelligence (AI) models and enabling machines in interpreting text, video, image or audio data. It also enables AI to learn and adapt. Hence, high quality AI training data is necessary for training AI models for producing results that are bias-free, representative and successful.
Data annotation is not only necessary in model training, but also plays a key role in the wider quality control process with respect to data collection. Annotated datasets set the gold standard for measuring the model performance and quality of remaining datasets. It enables deployment of AI models in different applications including chatbots, speech recognition and automation. This in turn leads to performance optimization and dependable results. For instance, a self-driving car is reliant on data it receives from computer vision, natural language processing, and sensors for making accurate driving decisions. To enable it to make distinction between roadblocks like other vehicles, people, animals, etc, the data has to be labeled or annotated.
What are the tools used in Data Annotation?
There are various tools and technologies used in data annotation. These range from simple manual annotation software to sophisticated technologies offering semi-automated and fully-automated annotation functions.

Manual Annotation Tools: These are software applications that permit human annotators to perform data labeling by hand. Interfaces are offered by these tools for performing tasks like drawing bounding boxes, segmenting images, and labeling objects within images. Examples of these tools include LabelImg, VGG Image Annotator (VIA), LabelMe, etc.
Semi-automated Annotation Tools: This tool is open sourced and provides automated annotation through pre-trained models to help with annotation. Examples of these tools include CVAT (Computer Vision Annotation Tool), MakeSense.ai, etc.
Automated Annotation Tools: This tool is fully automated and its main objective is to get rid of the need for human intervention by using advanced AI models for producing annotations. It hastens the annotation process, however, their efficacy depends on the complexity of the task along with the quality of the pre-existing data. Examples of these tools include proprietary systems developed by AI research labs and companies which are customized for particular use cases or datasets.
What are the types of Data Annotation (Text, Audio, Video)?
Data annotation can be performed on image, text, audio and video. The different types of annotations are as follows:
- Image labeling: Tags or labels are assigned to an entire image to describe its content. It is frequently used in categorizing tasks where the model learns classification of images as per the prescribed labels.
- Bounding boxes: Rectangular labels are drawn around objects inside an image for specifying its location and boundaries. This is critical for object detection models as it enables them to identify and pinpoint objects in various contexts.
- Segmentation: It divides an image into segments or pixels belonging to various objects or classes. It consists of two main types; semantic segmentation and instance segmentation. Semantic segmentation involves labeling each pixel of an image with a class of the object that it belongs to and doesn’t differentiate between independent objects of the same class. Instance segmentation differentiates between single objects of the same class.
What are the Benefits of Data Annotation?
- Quality and Accuracy: Accurate annotations assist AI models in understanding the slightest differences among objects in various contexts. This enables predictions or decisions which are dependable based on visual inputs.
- Model Training: Data annotation tutors models to recognize and comprehend different patterns, shapes, and objects. It offers them with examples to learn from. While accurate annotations result in more precise and reliable models, poor annotations limit a model’s capability in making correct identifications or predictions.
- Model Performance and Reliability: This is directly linked to the quality of the annotated data your AI model is trained on. AI models trained on annotated data are better able to handle the nuances and variability of real-world visual data. This leads to greater accuracy and reliability in its output which is critical in applications like medical diagnosis, autonomous driving, surveillance, etc.
- Innovation and Application: Data annotation plays a key role in driving innovation. It assists researchers and developers in pushing the boundaries with regard to what computer vision can achieve, explore new applications and improve existing ones. Data annotation allows for developing sophisticated models for advancements in AI and machine learning for transforming industries and improving lives.
What are the Challenges in Data Annotation?
- Scale and Complexity: With the rising demand for sophisticated and versatile AI systems, there’s a requirement for extensive, well-annotated datasets encompassing a broad range of situations and variations.Annotation of large datasets is not just time-intensive, but also needs precision for ensuring data quality. Also, the complex nature of particular images with objects occluded, partially visible or showcased in poor lighting conditions adds to the difficulty.
- Subjectivity and Consistency: Data annotation involves a great degree of subjectivity with respect to tasks that require identification of nuanced or abstract features within an image. Various annotators have varying interpretation of the same image resulting in inconsistencies in data which might impact the training of computer vision models that rely on consistent data for learning the way in which they can accurately recognize and interpret visual information. Hence, in order to maintain accuracy, consistency must be ensured across vast volumes of data along with clear guidelines and quality control measures.
- Cost and Quality: Data annotation gets very costly as high levels of accuracy are required. Manual annotation ensures high quality data but is labor intensive and costly. Automated annotation can result in lowered costs with increase in annotation speed, however the accuracy levels will vary. Hence, it is prudent to invest in a combination of both to limit the above challenges. Moreover, planning and careful consideration is needed for ensuring efficacy of the resultant models.
Summing up, it can be said that data annotation plays an indispensable role in the development of computer vision technologies.
Discover more from reviewer4you.com
Subscribe to get the latest posts to your email.