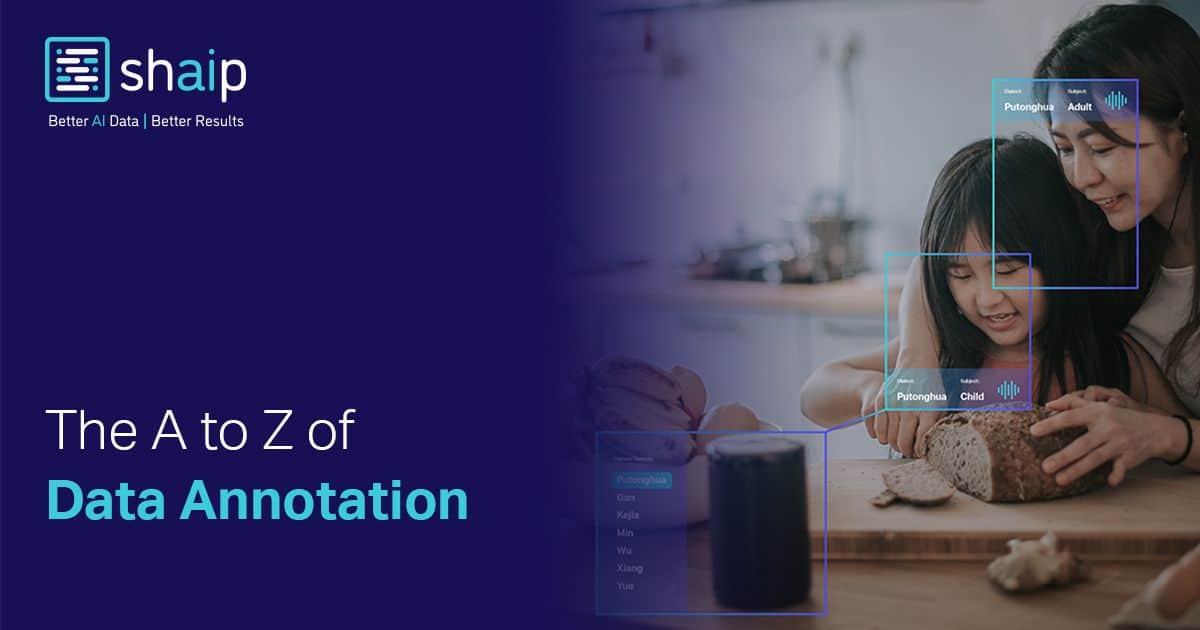
Your Goal
The first element you need to define is the goal with your artificial intelligence and machine learning concepts.
- Why are you implementing them in your business?
- Do they solve a real-world problem your customers are facing?
- Are they making any front-end or backend process?
- Will you use AI to introduce new features or optimize your existing website, app or a module?
- What is your competitor doing in your segment?
- Do you have enough use cases that need AI intervention?
Answers to these will collate your thoughts – which may currently be all over the place – into one place and give you more clarity.
AI Data Collection / Licensing
AI models require only one element for functioning – data. You need to identify from where you can generate massive volumes of ground-truth data. If your business generates large volumes of data that need to be processed for crucial insights on business, operations, competitor research, market volatility analysis, customer behavior study and more, you need a data annotation tool in place. However, you should also consider the volume of data you generate. As mentioned earlier, an AI model is only as effective as the quality and quantity of data it is fed. So, your decisions should invariably depend on this factor.
If you do not have the right data to train your ML models, vendors can come in quite handy, assisting you with data licensing of the right set of data required to train ML models. In some cases, part of the value that the vendor brings will involve both technical prowess and also access to resources that will promote project success.
Budget
Another fundamental condition that probably influences every single factor we are currently discussing. The solution to the question of whether you should build or buy a data annotation becomes easy when you understand if you have enough budget to spend.
Compliance Complexities
Vendors can be extremely helpful when it comes to data privacy and the correct handling of sensitive data. One of these types of use cases involves a hospital or healthcare-related business that wants to utilize the power of machine learning without jeopardizing its compliance with HIPAA and other data privacy rules. Even outside the medical field, laws like the European GDPR are tightening control of data sets, and requiring more vigilance on the part of corporate stakeholders.
Manpower
Data annotation requires skilled manpower to work on regardless of the size, scale and domain of your business. Even if you’re generating bare minimum data every single day, you need data experts to work on your data for labeling. So, now, you need to realize if you have the required manpower in place.If you do, are they skilled at the required tools and techniques or do they need upskilling? If they need upskilling, do you have the budget to train them in the first place?
Moreover, the best data annotation and data labeling programs take a number of subject matter or domain experts and segment them according to demographics like age, gender and area of expertise – or often in terms of the localized languages they’ll be working with. That’s, again, where we at Shaip talk about getting the right people in the right seats thereby driving the right human-in-the-loop processes that will lead your programmatic efforts to success.
Small and Large Project Operations and Cost Thresholds
In many cases, vendor support can be more of an option for a smaller project, or for smaller project phases. When the costs are controllable, the company can benefit from outsourcing to make data annotation or data labeling projects more efficient.
Companies can also look at important thresholds – where many vendors tie cost to the amount of data consumed or other resource benchmarks. For example, let’s say that a company has signed up with a vendor for doing the tedious data entry required for setting up test sets.
There may be a hidden threshold in the agreement where, for example, the business partner has to take out another block of AWS data storage, or some other service component from Amazon Web Services, or some other third-party vendor. They pass that on to the customer in the form of higher costs, and it puts the price tag out of the customer’s reach.
In these cases, metering the services that you get from vendors helps to keep the project affordable. Having the right scope in place will ensure that project costs do not exceed what is reasonable or feasible for the firm in question.
Open Source and Freeware Alternatives
Some alternatives to full vendor support involve using open-source software, or even freeware, to undertake data annotation or labeling projects. Here there’s a kind of middle ground where companies don’t create everything from scratch, but also avoid relying too heavily on commercial vendors.
The do-it-yourself mentality of open source is itself kind of a compromise – engineers and internal people can take advantage of the open-source community, where decentralized user bases offer their own kinds of grassroots support. It won’t be like what you get from a vendor – you won’t get 24/7 easy assistance or answers to questions without doing internal research – but the price tag is lower.
So, the big question – When Should You Buy A Data Annotation Tool:
As with many kinds of high-tech projects, this type of analysis – when to build and when to buy – requires dedicated thought and consideration of how these projects are sourced and managed. The challenges most companies face related to AI/ML projects when considering the “build” option is it’s not just about the building and development portions of the project. There is often an enormous learning curve to even get to the point where true AI/ML development can occur. With new AI/ML teams and initiatives the number of “unknown unknowns” far outweigh the number of “known unknowns.”
Build | Buy |
---|---|
Pros:
|
Pros:
|
Cons:
|
Cons:
|
To make things even simpler, consider the following aspects:
- when you work on massive volumes of data
- when you work on diverse varieties of data
- when the functionalities associated with your models or solutions could change or evolve in the future
- when you have a vague or generic use case
- when you need a clear idea on the expenses involved in deploying a data annotation tool
- and when you don’t have the right workforce or skilled experts to work on the tools and are looking for a minimal learning curve
If your responses were opposite to these scenarios, you should focus on building your tool.
Discover more from reviewer4you.com
Subscribe to get the latest posts to your email.